How Effective is the Automation and Validating of Human Dose Predictive Models?
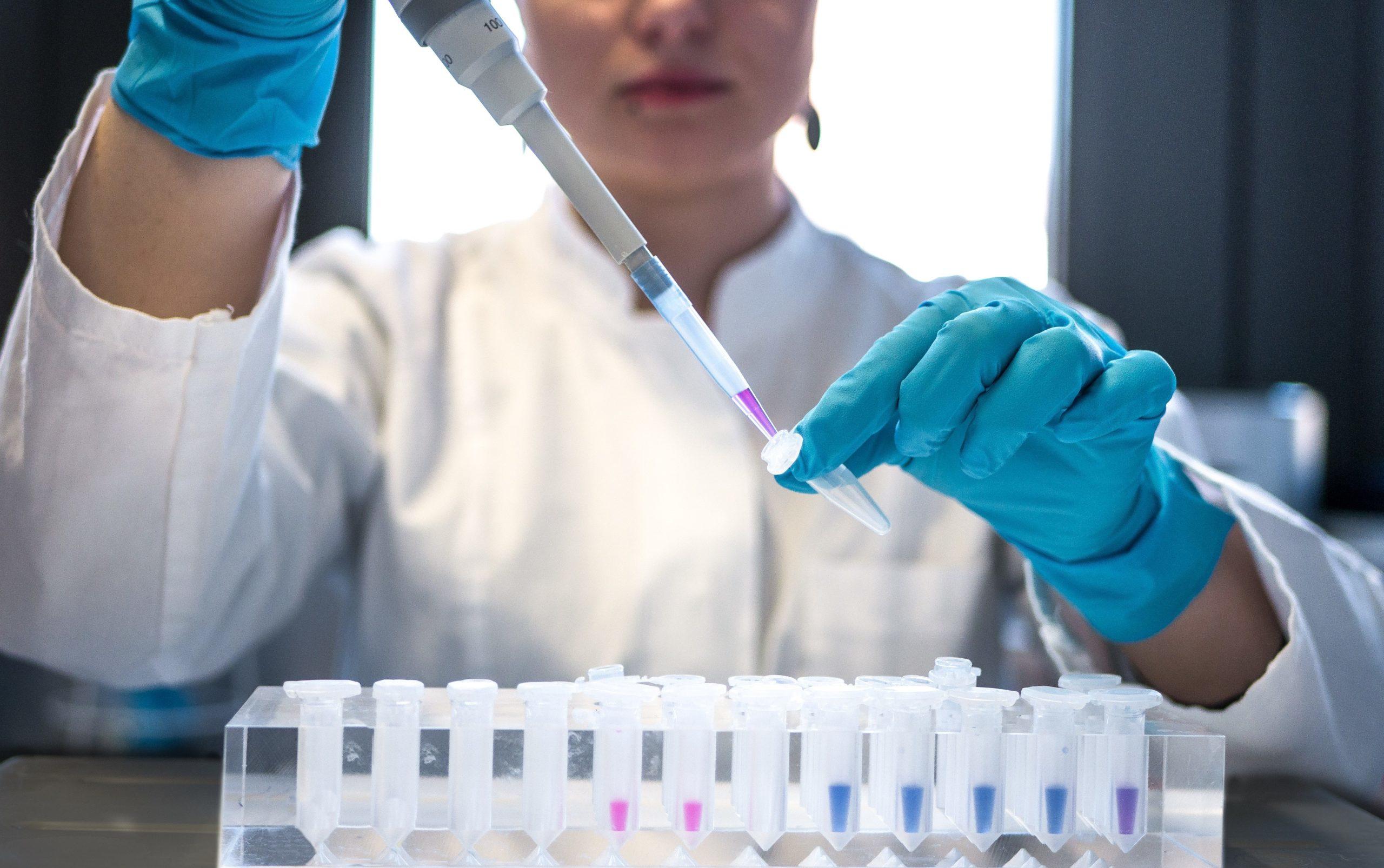
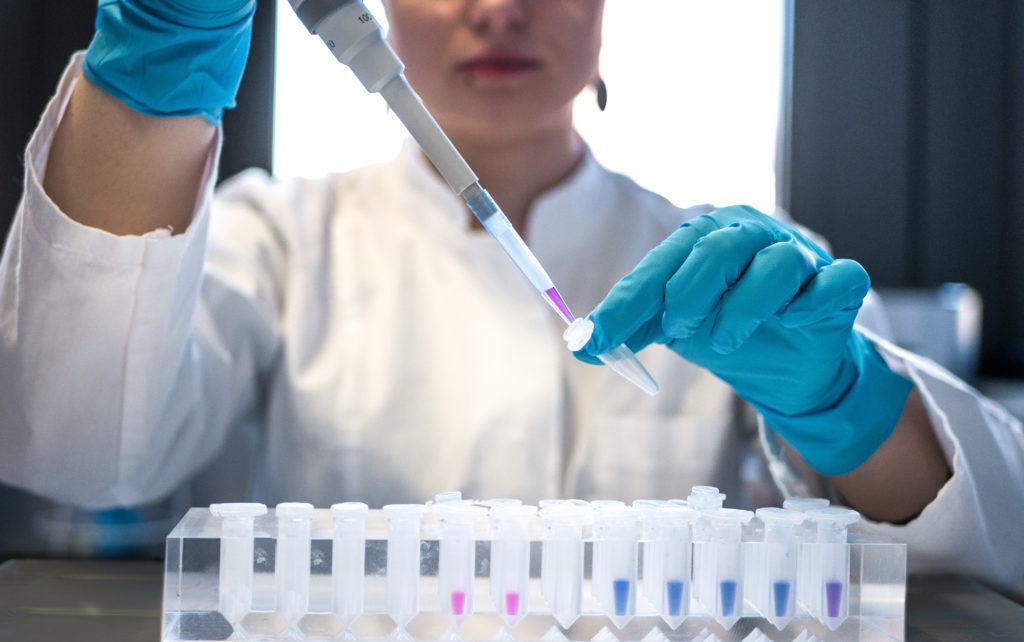
Dose prediction is a critical part of drug discovery which determines the safety and efficacy of drugs within patients. Dose response predictions are made with preclinical models which quantify pharmacokinetic (PK) behaviour via a number of parameters. Despite the continuous use of in vitro and in vivo models, it appears that automated, machine learning approaches are leading the way in the latest innovations of human dose prediction.
For daily articles on the latest pharma trends and innovations, as well as interviews with leading experts and in-depth industry White Papers, subscribe to PharmaFeatures.com.
Introduction
There are two main factors linked to dose prediction: PK and drug-drug interactions. Pharmacokinetics is the behaviour of a drug within a system, and includes bioavailability, distribution, metabolism and excretion. Drug-drug interactions (DDI) can occur when multiple drugs are co-administered to a patient. DDI is an important part of drug development, as it can lead to altered systemic exposure, causing variable drug responses. Typically DDIs occur due to inhibition of the metabolism for one drug by the other; it leads to a rise in plasma concentration of the drug whose metabolism is inhibited.
Preclinical data helps to inform of the likelihood of achieving the desired therapeutic dose in early clinical development. From here, the prediction methods are assessed and further refined from the comparison of clinical data with the original predictions. Due to continuous developments in predictive methodologies and data analysis, the probability of successful PK predictions has increased over the past few years. According to a 2020 study, 83% of AstraZeneca drug development projects progress in the clinic with no PK issues.
The higher the probability of successful predictive models, the faster the progression from drug discovery to market. Poor prediction of drug behaviour in clinical trials can significantly hinder drug development, either due to poor efficacy or toxicity issues. In terms of safety testing, drugs are often tested in concentration-response assays. The results are quantified by an XC50 value, which is a measure of the potency of the compound, and which lies between the lowest and highest concentrations tested.
Potency is a key component of predicting PK and is a measure of drug activity relative to the amount of drug required to produce a desired effect. Another important assessment is the maximum concentration of a compound when it reaches the target, known as Cmax. This refers to the clinical dose, and compounds given at higher doses tend to have a greater risk of toxic side effects, making Cmax one of the best predictors of clinical toxicity.
Efficacy of current models
Through a combination of in silico, in vitro and in vivo assays, there are four PK parameters a drug must pass in order to inform about its pharmacokinetic activity for dose prediction.
Prediction of volume distribution (Vd) is a measure of the relative affinity of a drug for tissues and plasma. Vd is reasonably predicted from in silico and in vitro modelling. Using preclinical in vivo PK data, typically animal models, Vd is the most predictable of the PK parameters.
The prediction of drug clearance (CL) uses a combination of animal data and human in vitro modelling. The primary CL routes in humans and preclinical species are hepatic metabolism and renal and biliary elimination, and methods are required to predict these parallel elimination processes in humans. Identifying the elimination route and rate in preclinical species relative to human CL makes this one of the most challenging parameters in drug discovery.
Allometry is a method used to predict drug CL and is still widely used within the industry. According to a 2016 study, allometric scaling is where the “exchange of drug dose is based on normalization of dose to body surface area”. In other words, it is used to scale physiological rates relative to the growth and size of one (animal) species relative to another.
Prediction of bioavailability is another key parameter and refers to the extent and rate at which a drug accesses the target site. Poor bioavailability comprises the efficacy of a drug if the desired therapeutic dose is not correct once the drug reaches its target. In addition, low bioavailability can create issues in obtaining appropriate exposures in pharmacodynamic (PD), efficacy, and safety experiments for a candidate drug.
Finally, the prediction of pulmonary absorption is an important part of optimising drugs delivered directly to the lungs. The two main strategies for optimising drug retention in the lungs after inhalation involves maintaining a drug reservoir within the airways and a slow rate of dissolution.
Innovations in predicting human dose
The industry has seen continuous developments in in vitro/vivo/silico models in order to optimise human dose production. However it appears that computational biology and AI are leading the way in the latest innovations.
In immunology, a 2021 study used machine learning (ML) attempts for predicting human subcutaneous bioavailability of monoclonal antibodies. The aim of this study was to develop a ML approach to determining whether the bioavailability of monoclonal antibodies was >70%. The measured bioavailability of the monoclonal antibodies ranged from 35% to 90%. The team used multiple ML methods including random forest and decision trees. These tree-based methods proved to best predict bioavailability.
Since all of the ML approaches used theoretical calculations and predictions for input, it was suggested from the study that these models may be most useful for early-stage activities like molecule formational design.
Oncology is another therapeutic area which has utilised automated methods for dose prediction. Intensity modulated radiation therapy is a deep learning approach which delivers specific doses to target tissue while sparing adjacent organs at risk. This is an important part of cancer treatment, by helping to reduce radiation-related toxicities.
In 2020, a study investigated fully automated dose prediction using generative adversarial networks in prostate cancer patients. The preliminary study focused on CTs of prostate cancer patients using a generative adversarial network framework, the choice of which was suggested to be due to the “variations of the targets in the prostate cancer patients [being] relatively small”.
The future for ML methods is suggested to optimise treatment strategy before radiation, as radiotherapy can cause complications with organ placement and re-irradiation.
To discuss these topics further with sector experts, and to ensure you remain up-to-date on the latest in clinical development, sign up for Proventa International’s Medicinal Chemistry and Biology Strategy Meeting, set for 29 June 2021.
Charlotte Di Salvo, Junior Medical Writer
Proventa International
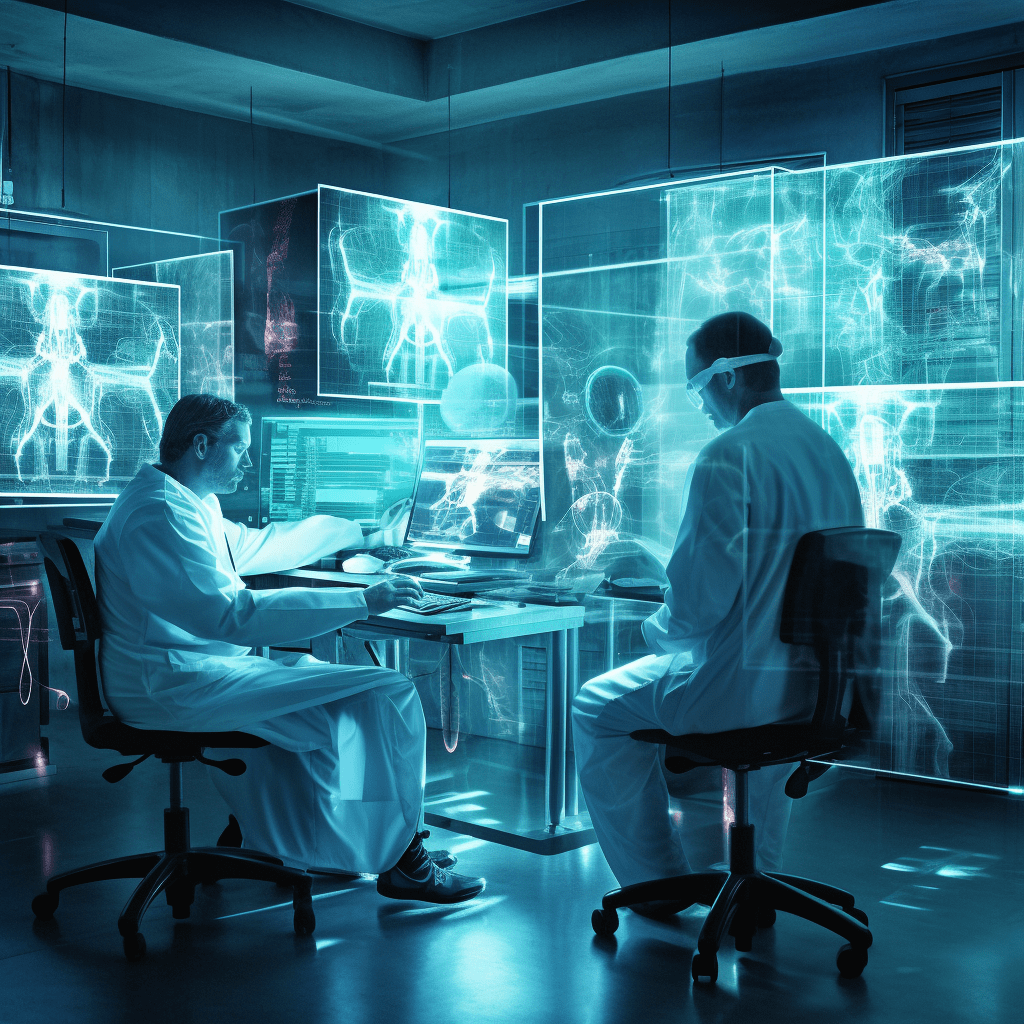
Navigating the Complex World of Global Regulatory Affairs in Oncology
In today's fast-paced global pharmaceutical landscape, the regulatory affairs sector plays a pivotal role in ensuring the safety, efficacy, and market access of oncology drugs. As the demand for innovative cancer therapies continues to grow, understanding the intricacies of global...
2 years agoNavigating the Complex World of Global Regulatory Affairs in Oncology
In today's fast-paced global pharmaceutical landscape, the regulatory affairs sector plays a pivotal role in ensuring the safety, efficacy, and market access of oncology drugs. As the demand for innovative cancer therapies continues to grow, understanding the intricacies of global...
2 years ago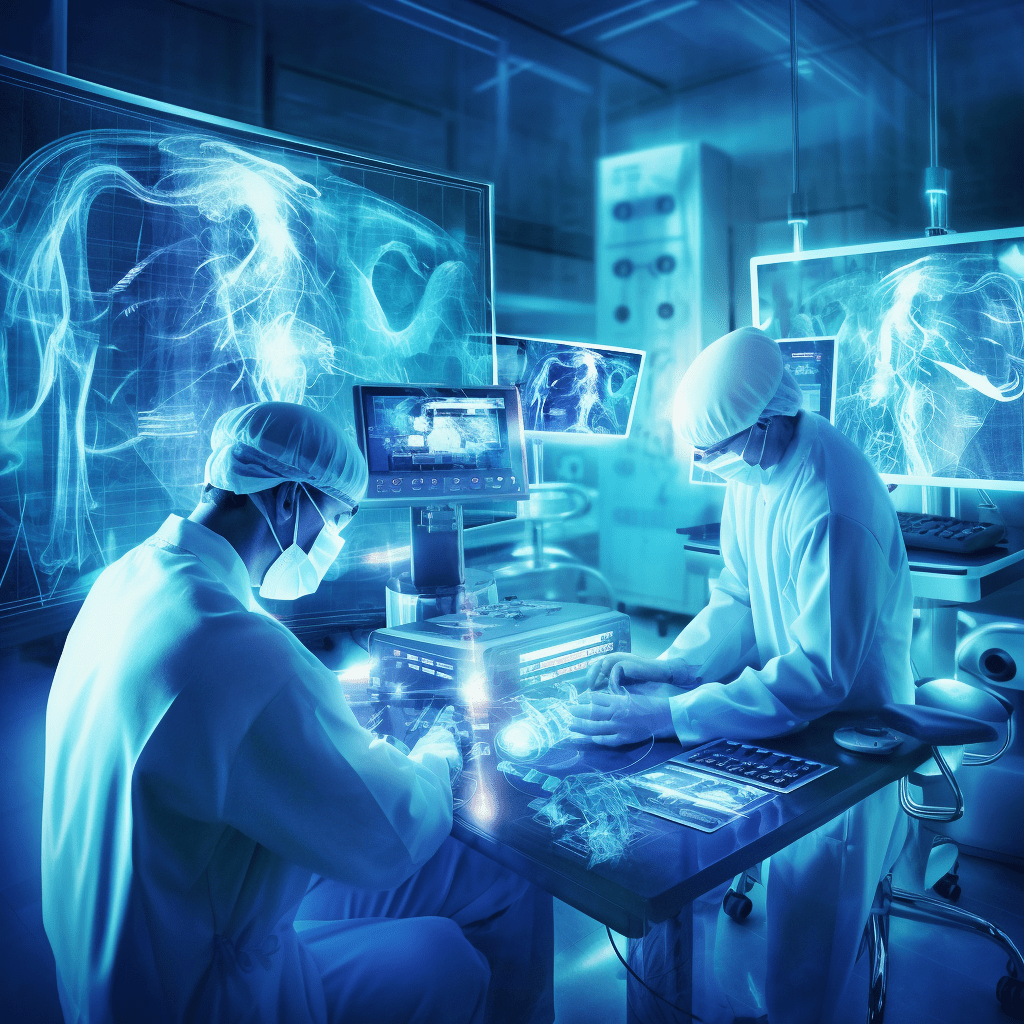
Overcoming the Hurdles: Navigating the Challenges in Oncology Clinical Trials
In the world of medical research, oncology clinical trials are at the forefront of innovation and discovery. These trials play a crucial role in advancing our understanding of cancer and developing more effective treatments. However, the path to successful oncology...
2 years agoOvercoming the Hurdles: Navigating the Challenges in Oncology Clinical Trials
In the world of medical research, oncology clinical trials are at the forefront of innovation and discovery. These trials play a crucial role in advancing our understanding of cancer and developing more effective treatments. However, the path to successful oncology...
2 years ago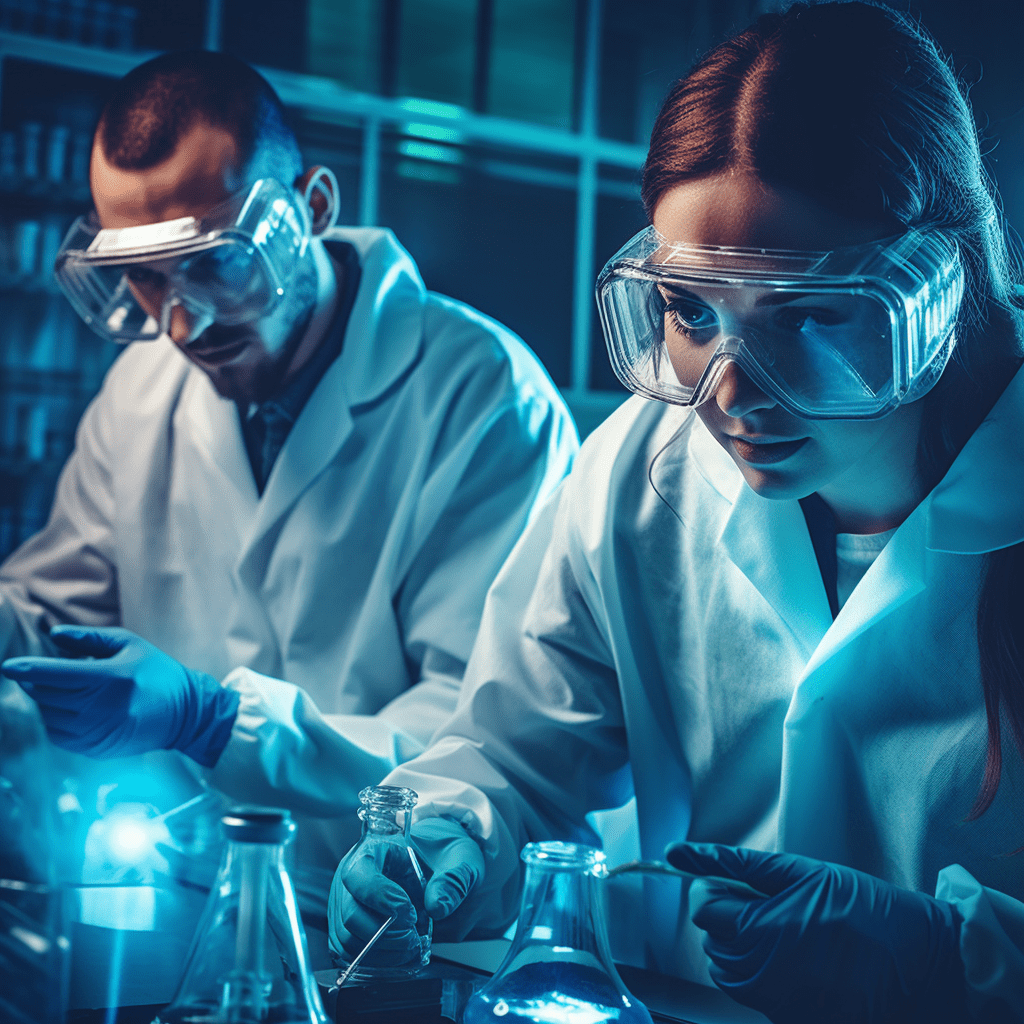
Embracing a Patient-Centric Approach in Oncology Trials
In the realm of healthcare and medical research, the term "patient-centric" has gained significant traction in recent years. This shift in focus towards prioritizing patients' needs and preferences is not only transforming the healthcare industry but is also making waves...
2 years agoEmbracing a Patient-Centric Approach in Oncology Trials
In the realm of healthcare and medical research, the term "patient-centric" has gained significant traction in recent years. This shift in focus towards prioritizing patients' needs and preferences is not only transforming the healthcare industry but is also making waves...
2 years ago