Machine Learning Data Analysis: Applications Across Immunology, Oncology and Diabetes
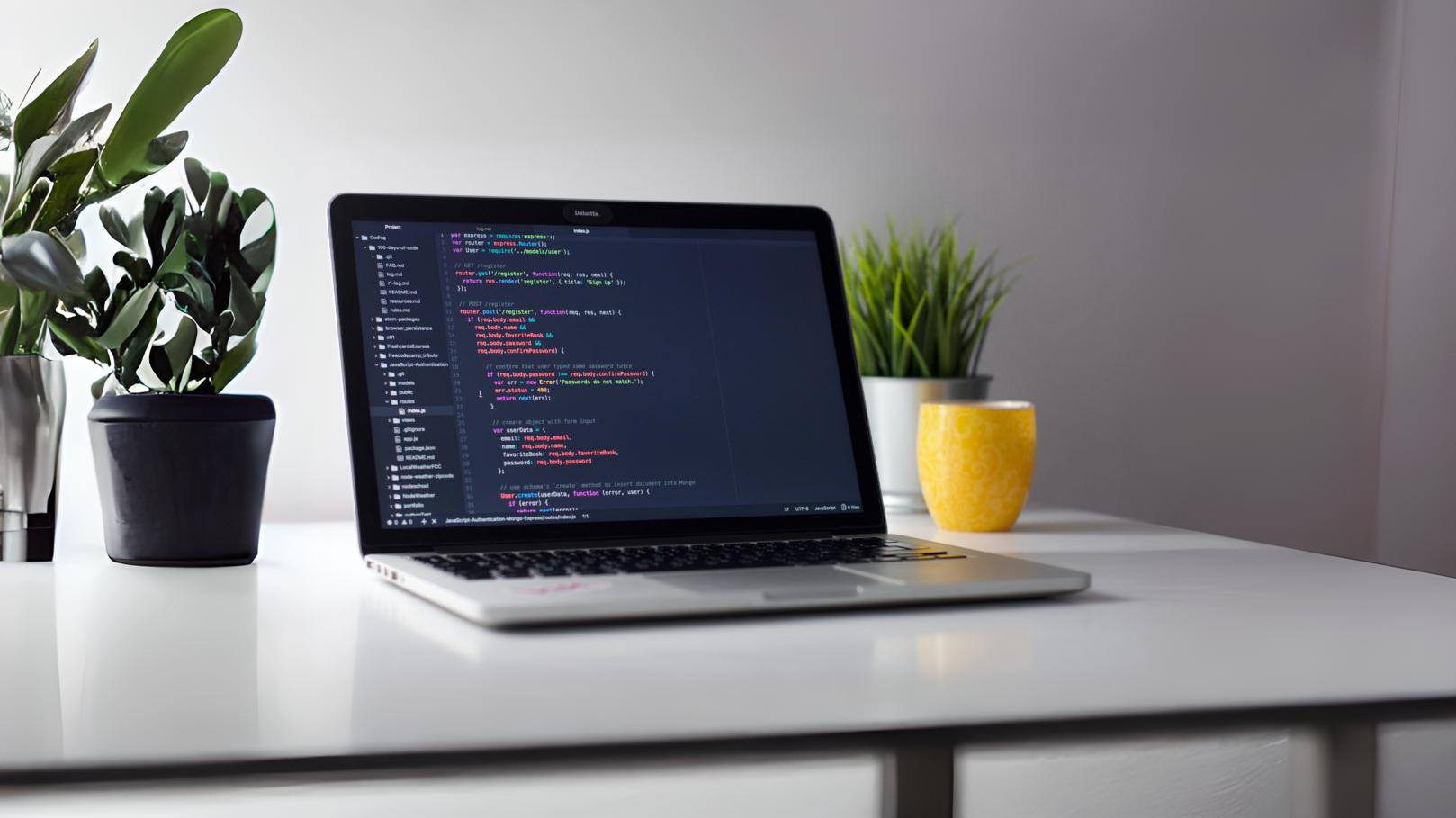
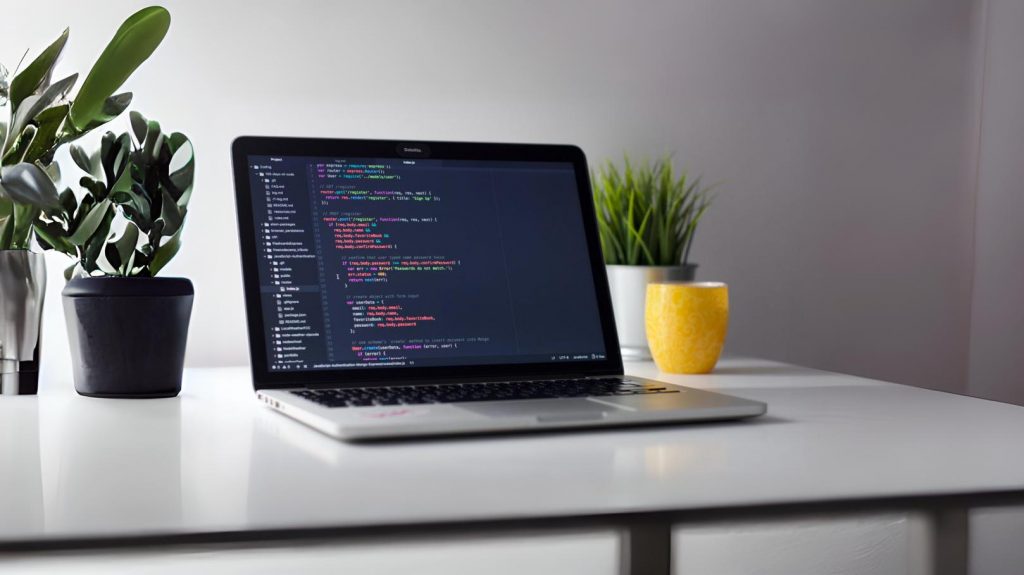
Computational modelling is becoming increasingly popular for data analysis in life sciences. Vast areas of therapeutic research are taking advantage of machine learning (ML) approaches for disease predictions and pathology. Cancer image analysis and diabetes case prediction are a few of the latest innovations.
For daily articles on the latest pharma trends and innovations, as well as interviews with leading experts and in-depth industry White Papers, subscribe to PharmaFeatures.com.
Hear from some of the industry leaders including Steven Zimmer who will be providing his expertise in leading a discussion on how multi-targeted drugs could be the solution to low drug discovery productivity. To discuss these innovations and more with other leading experts in an informal setting, sign up to Proventa’s Bioinformatics Strategy Meetings, held online on 1 July 2021.
ML is a branch of artificial intelligence showing exciting applications across drug development. With each exposure to new data, an ML machine-learning algorithm grows increasingly better at recognising patterns over time. There are two main techniques used to apply ML: supervised and unsupervised learning. Unsupervised learning is a type of algorithm that learns patterns from data without tags (annotations). Supervised learning algorithms, on the other hand, are formed from labelled training data which consists of a set of training examples. In others words, supervised learning relies on human intervention to label data in order to train the model to search for a specific component – cancer image analysis for example. Unsupervised learning on the other hand, analyses vast amounts of data which has not been labelled in order to identify associations or trends.
Supervised learning methods have been used to predict future values of data categories or continuous variables. Unsupervised learning is primarily used for exploratory purposes in the development of models to enable data clustering in a format not specified by the user. This particular technique helps to identify hidden patterns within input data, whereas supervised learning methods predict future outputs based on a trained model of known input and output data.
Diabetes research
In March 2020, an abstract supplement was published detailing the “development of a machine-learning method for predicting new onset of diabetes mellitus (DM)”. While data predictions are not a novel concept within diabetes research, they typically only apply for those predisposed to health conditions, not healthy individuals.
Within this study, an ML-based prediction model was used to identify DM signatures prior to onset. Signatures for DM could be biomarkers, for example, or blood-based factors like serum proteins.
These signatures would be identified through the data analysis of nationwide health records of patients from 2008-2018 via the ML prediction-based model. The model utilised a type of ML known as gradient-boosting decision trees. A gradient-boosting decision tree (GBDT) model is typically a prediction-based form of AI used to calculate the likelihood of interactions.
The study identified a total of 4,696 new diabetes patients (7.2%) from datasets. Their ML model predicted the future incidence of diabetes with an overall accuracy of 94.9%.
It is worth noting however that the algorithm for GBDT was originally developed for static data, i.e. fixed size of data. However, in the context of diabetes research, data would be constantly changing with incoming patient medical records. Therefore, it could potentially be a time-consuming and impractical process to run GBDT every time incoming data arrives. In terms of solutions, one article has suggested that GBDT needs to be adapted to “an incremental learning setting, where new samples are continuously arriving in batches”.
Diabetes mellitus is a chronic disease and increases the risk of developing diseases such as cancer and atrial fibrillation, which can be fatal. Hence, predicting diabetes in the population could prevent potential cases through medication or diet control. In the long-term, this would reduce the likelihood of said patients developing serious diseases as a result of diabetes, which would theoretically reduce the pressure on healthcare systems around the globe.
Immunology
Artificial intelligence has been used as an important tool within immunology to answer highly complex questions. More recently, a study published August 2020 demonstrated that deep learning neural networks can be used to differentiate between immune cells. They specifically used a convolutional neural network (CNN), a form of deep learning. A CNN is a deep learning model inspired by the “animal visual cortex in structure and designed to automatically and adaptively learn spatial hierarchies of features, from low- to high-level patterns”.
The study demonstrated how this ML approach could learn to predict the patterns of chromatin opening across 81 stem and differentiated cells across the immune system, solely from the DNA sequence of regulatory regions.
Chromatin is the material which constitutes a chromosome composed of DNA and protein.
Open chromatin regions reflect quite closely gene expression in the corresponding cells, hence why these areas are a target for cell identification in the immune system.
This deep learning approach has shown to be an important tool for immunology researchers, revealing modalities and complex patterns of immune transcriptional regulators that arise directly from the DNA sequence. Immune transcriptional regulators play a critical role in the maintenance of the immune system. These factors primarily control gene expression for various immune cells, thus have been implicated in autoimmune disorders when the immune system malfunctions.
This was raised in a 2018 study which emphasised how dysregulated (gene) expression has been correlated to immune cell dysfunction in autoimmunity and lymphomagenesis. Therefore ML approaches like those used in the aforementioned study may help researchers to understand the complex mechanism behind cellular phenotype in the immune system. And, potentially, contribute to therapeutic developments for many immunological disorders.
Oncology
In addition to immunology, deep learning approaches in oncology are becoming increasingly popular across basic and clinical cancer research.
Deep learning approaches have brought significant advancements to cancer image analysis. Early-stage cancer is often difficult to detect, especially so with conventional technology and human error, thus ML approaches like convolutional neural networks could potentially analyse images with greater speed and accuracy.
There are a number of challenges, however, with the deep learning approach to image analysis. Firstly, differences in colour tone on pathology slides may occur across different institutions due to the type of staining and sample preparation protocols: i.e. it presents an issue if one research lab uses colour x to stain their samples to highlight cancerous regions but the ML model has been trained from images of samples stained with Y, it could be difficult to accurately detect cancer as it is not the same staining. Therefore, it is necessary to “standardize color tones in digital slides for the development of accurate AI algorithms”.
Secondly, the limited number of medical images available for network training is a problem. Data augmentation is one strategy that has been developed by researchers to overcome this issue. Data augmentation i.e. where images are randomly cropped, tilted, inverted or flipped to increase their number, is one effective strategy for dealing with the small size of the training set.
A 2017 study successfully trained a CNN to classify skin cancer with a level of competence comparable to dermatologists. Using only pixels and disease labels as inputs, they classified skin lesions via a single CNN. The 1.4 million pre-training and training images in this study overcame photographic variability like zoom and lighting.
This is a huge step forward for cancer imaging. The development of an accurate ML model for image analysis could support medical practitioners and patients to “proactively track skin lesions and detect cancer earlier”. Early detection significantly impacts cancer prognosis for many patients and MLapproaches like this could save many lives.
To discuss these topics further with sector experts, and to ensure you remain up-to-date on the latest in clinical development, sign up for Proventa International’s Bioinformatics Strategy Meeting, set for 1 July 2021.
Charlotte Di Salvo, Junior Medical Writer
Proventa International
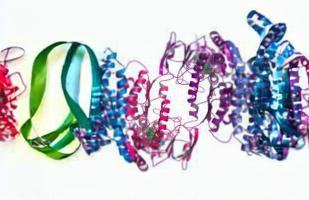
DeepMind’s AI Predicts Structures for More Than 350,000 Proteins
In 2003, researchers sequenced approximately 92% of the human genome, a huge achievement and very recently researchers have completed the entire process. Now, the latest innovation in AI technology has predicted the structure of nearly the entire human proteome. The...
4 years agoDeepMind’s AI Predicts Structures for More Than 350,000 Proteins
In 2003, researchers sequenced approximately 92% of the human genome, a huge achievement and very recently researchers have completed the entire process. Now, the latest innovation in AI technology has predicted the structure of nearly the entire human proteome. The...
4 years ago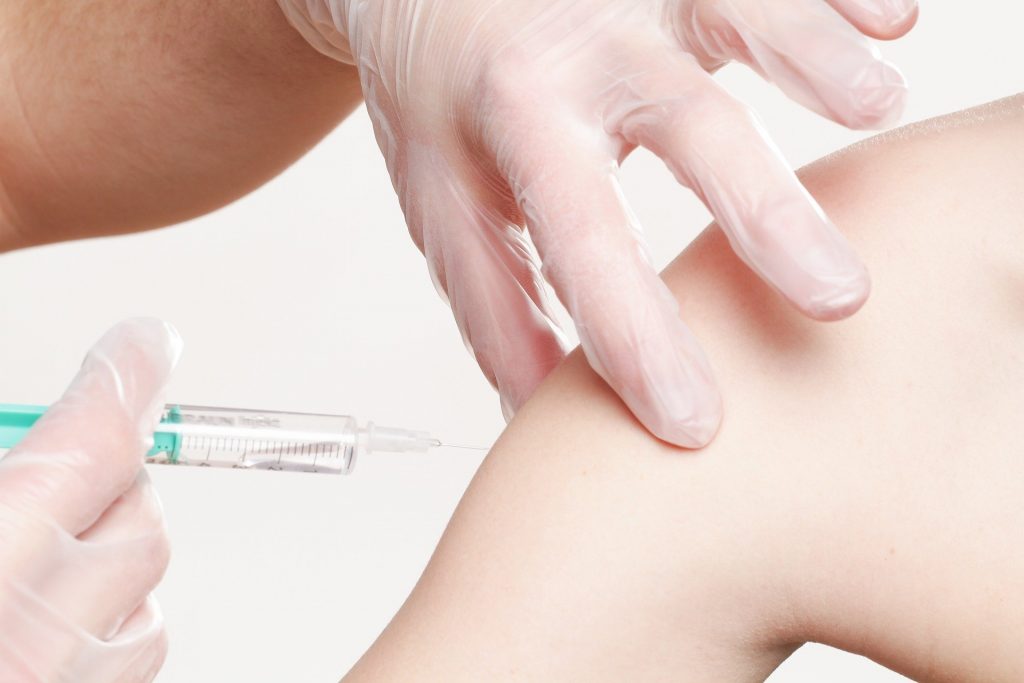
COVID-19 Booster Controversy: Disputes Across the Pharma Industry
Despite almost two thirds of the adult population in the UK having been vaccinated, cases are on the rise, which appear to be associated with the highly-transmissible delta variant of COVID-19. According to The European Centre for Disease Prevention and Control,...
4 years agoCOVID-19 Booster Controversy: Disputes Across the Pharma Industry
Despite almost two thirds of the adult population in the UK having been vaccinated, cases are on the rise, which appear to be associated with the highly-transmissible delta variant of COVID-19. According to The European Centre for Disease Prevention and Control,...
4 years ago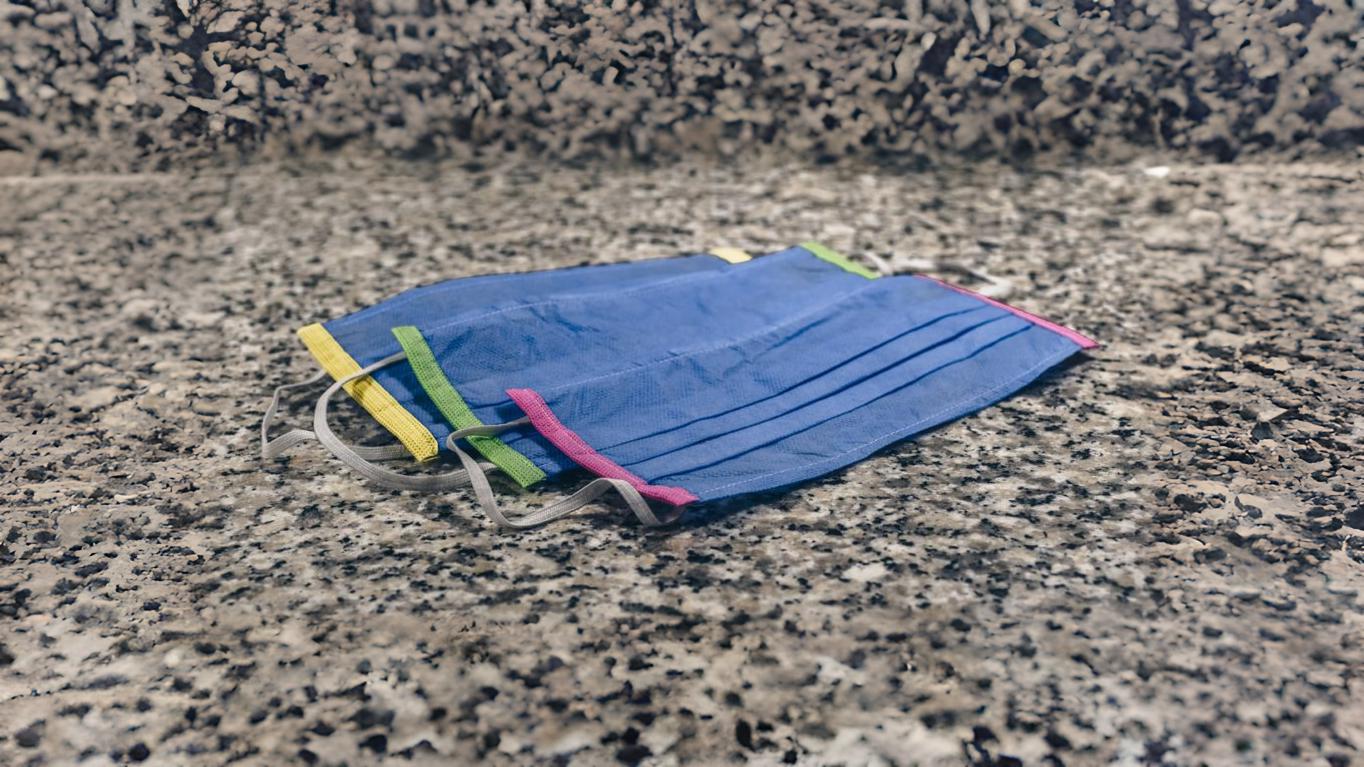
COVID’s Impact on Innovations in Regulatory Affairs
While the impact of COVID-19 was significant in every area of the pharmaceutical sector, some of the most pandemic’s most overlooked impacts were within the regulatory space. From study design to submissions, the virus affected every aspect of drug regulation,...
4 years agoCOVID’s Impact on Innovations in Regulatory Affairs
While the impact of COVID-19 was significant in every area of the pharmaceutical sector, some of the most pandemic’s most overlooked impacts were within the regulatory space. From study design to submissions, the virus affected every aspect of drug regulation,...
4 years ago