Innovations in AI Risk-Based Monitoring in Clinical Research
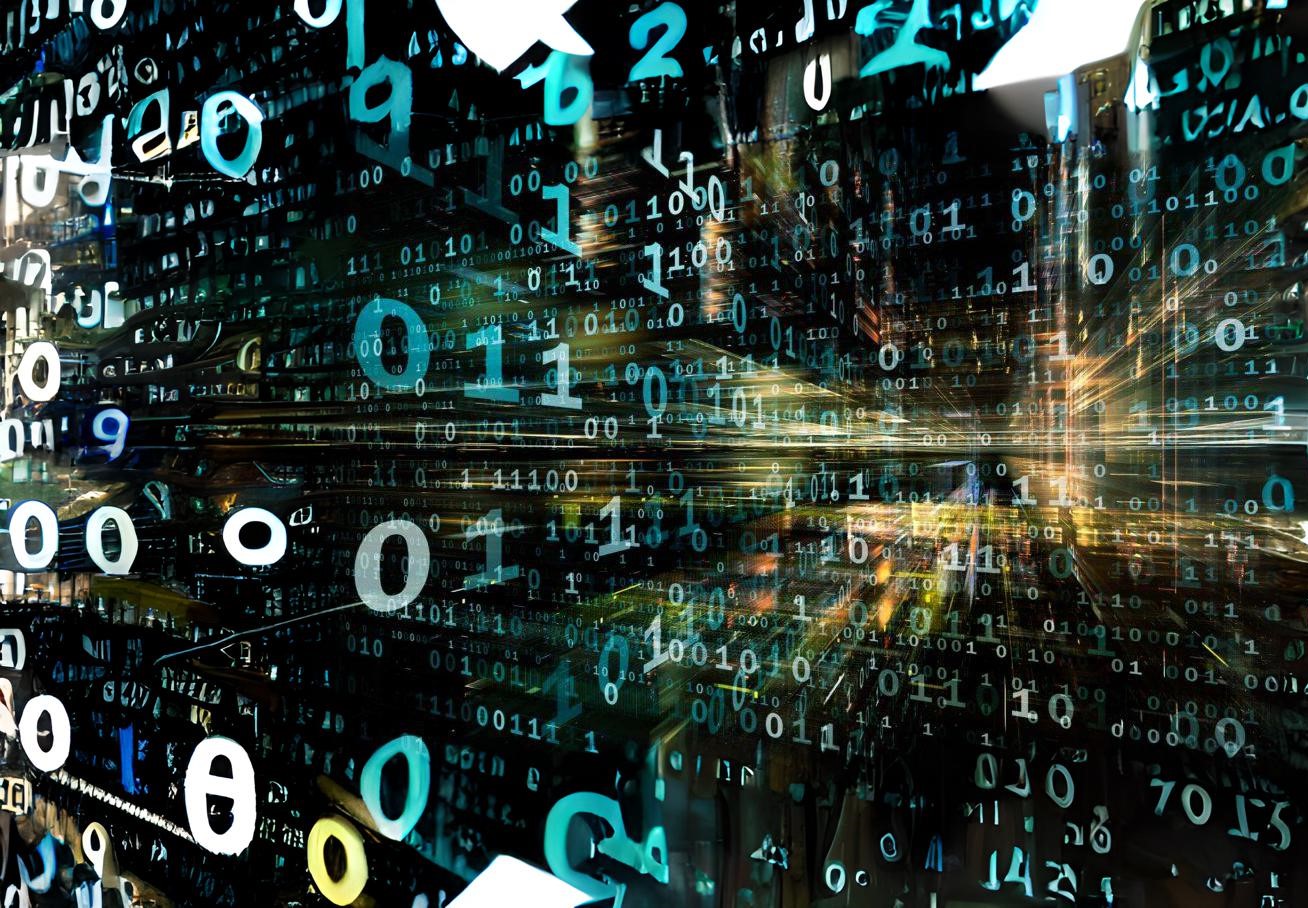
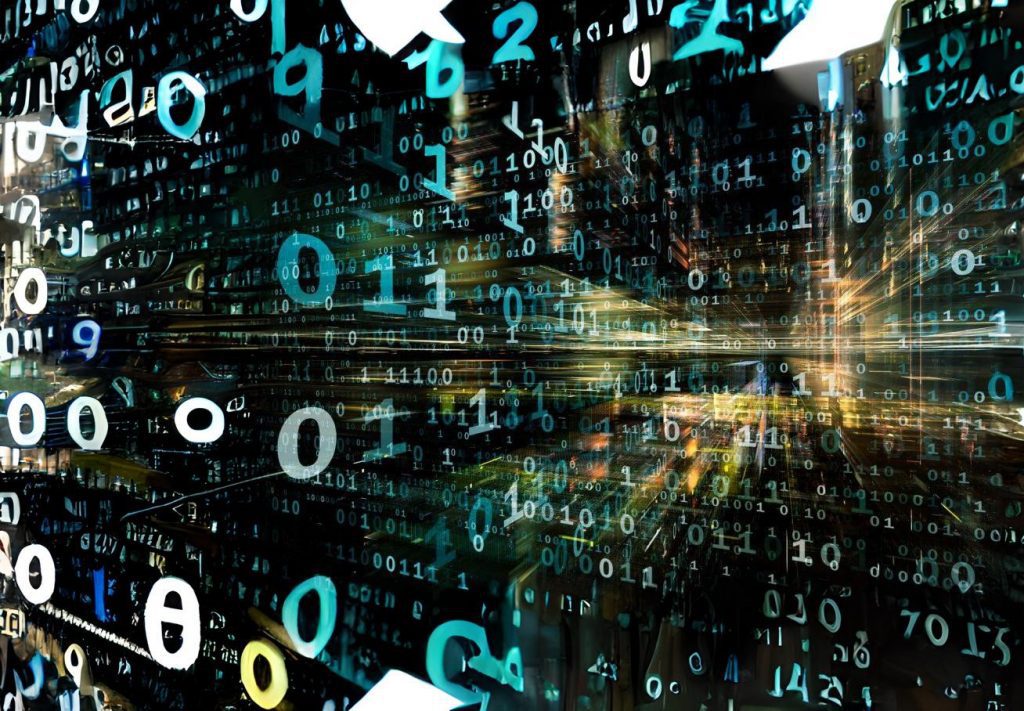
Risk-based monitoring (RBM) is a useful tool in maximising the quality of clinical trials. Enhanced site communication, greater patient safety and lower costs are a few of the benefits of RBM. The innovations of AI in clinical research have application in RBM, optimising further the design and conduct of clinical trials.
For daily articles on the latest pharma trends and innovations, as well as interviews with leading experts and in-depth industry White Papers, subscribe to PharmaFeatures.com.
RBM allows sponsors to identify and address potential issues that could comprise the quality of a clinical trial. Identifying, assessing, monitoring and minimising any potential risks is of paramount importance to prevent delay or termination of clinical trials.
There are three key parts of RBM:
• Identify critical data and processes
• Risk assessment
• Monitoring plan
According to FDA guidance, the risk assessment serves to “identify and understand the nature, sources, likelihood of detection, and potential causes of risks that could affect the collection of critical data or performance of critical processes”. The more information about high-risk areas for potential issues or critical data points, the easier a monitoring plan can be developed. In the FDA document, there are a number of points raised when determining the type of monitoring required. The complexity of study design; study endpoints; clinical complexity of study population; and geographic location of sites are four of the nine factors that need to be considered for RBM.
In addition to the obvious identification of a risk and its origin, re-education of the site and amendment to recruitment plans are other ways in which a risk assessment can be performed.
In order to identify the critical data points at the start of the clinical trial, it is important to identify the variables that need to be measured to answer the original scientific question of the study. Critical data points in clinical trials can include anything from the target parameters, to compliance for subject criteria to serious adverse events.
A white paper by IQVIA highlights a number of statistics in support of RBM for clinical trial efficiency, including “developed RBM solutions can bring as much as 25% cost reduction over traditional trial execution approaches”.
Application of AI in RBM
Predictive analytics
Predictive analytics is one of the more recent innovations in RBM that incorporates AI technology. It is the practice of extracting information from existing data in order to determine patterns and predict future outcomes and trends. In terms of risk management, it can be used to predict future scenarios with an acceptable level of reliability, in addition to ‘what-if’ scenarios which can be used to develop a risk assessment.
Machine learning (ML) is an important part of clinical predictive analytics. Due to the accessibility of electronic health records (EHR), the volume of data in clinical research has increased substantially. Therefore, computational software has become widely used as a more cost- and time-efficient method of analysing vast amounts of data.
In 2018, a retrospective, single-site study investigated the use of ML to identify high-risk surgical patients using automatically curated electronic health record data (Pythia). The first aim of the study was to develop a ML model that could analyse high-volume, high-quality data (to monitor care and patient outcome). The second was to promote the development of ML models that can be used to interpret clinical data, and support clinicians in identifying potential high-risk patients.
Random forest and extreme gradient boosted decision trees were a few of the ML methods used to predict the likelihood of post-surgical complications. In addition, a method known as Lasso penalized logistic regression was used due to the ability of the algorithms to quantify the importance of variables in a dataset. The study emphasised that “by providing model users with additional information about predictor weights, clinicians can glean insights into potential patient risk mitigation strategies”.
The positive results demonstrated the efficacy of utilising ML for predictive analytics. The 42 models used demonstrated “strong predictive performance”, with the random forests showing the greater. The prediction of high-risk patients in this study reinforces the translatability to RBM in clinical research. Using predictive analytics could help forecast potential issues which can be addressed and alter risk assessments accordingly.
AI Data Security
Thanks to the introduction of digital innovations like telehealth, the amount of data within clinical research is increasing substantially. Furthermore, the pandemic has seen a rapid rise in the number of decentralised clinical trials globally. This new era of virtual clinical research has brought a number of advantages, streamlining clinical trials with cloud-based software. However, with confidential data like EHRs being shared across multiple platforms, data security has become a priority within RBM.
Blockchain is an example of technology which has converged with AI and is becoming increasingly popular for data security within clinical trials. The traditional centralised server models are vulnerable to the single-point attack limitations and malicious insider attacks. However, blockchain technology spreads data across a large network of databases in replica copies, rather than a single store. The benefit of this is that stored data is less vulnerable to hacking or infringement. Furthermore, the incorporated verification ensures data is protected from unauthorised access. In terms of the convergence with AI, blockchain can provide privacy and trustworthiness, and AI utilises machine learning algorithms on the blockchain to achieve security, scalability and effectiveness.
It is worth noting however, blockchain technology still has some limitations. Expensive software, large storage and high bandwidth are a few of the challenges with blockchain which may be not suitable for the smaller pharmaceutical companies and contract research organisations.
With the help of AI, RBM will continue to evolve at the same pace as the digitalisation of clinical research. The value of predictive analytics has reinforced the importance of developed models to enhance RBM for clinical trials, reducing the time and cost spent addressing issues that could have been predicted earlier.
To discuss these topics further with sector experts, and to ensure you remain up-to-date on the latest in clinical development, sign up for Proventa International’s Clinical Operations Strategy Meeting, set for 15 June 2021.
Charlotte Di Salvo, Junior Medical Writer
Proventa International
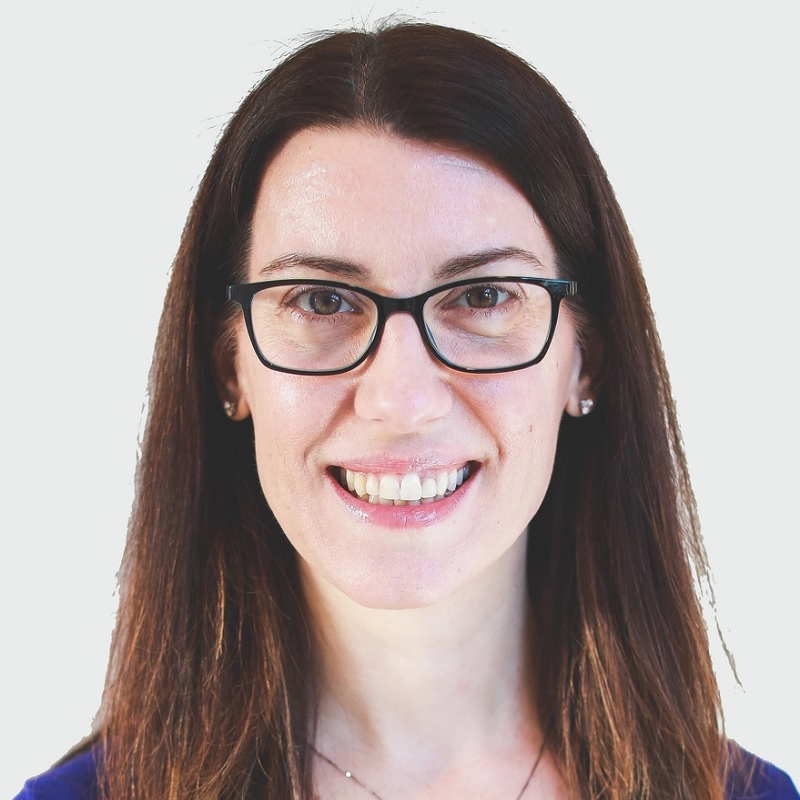
Raising Representation in Clinical Trials: An Interview with Liz Beatty, Inato
The COVID-19 pandemic created a significant shortage of suitable patients for clinical trials worldwide. Within this crisis was another more serious issue around inclusion and representation, driven by COVID’s disparate effect on certain ethnicities, that spoke to a wider issue...
4 years agoRaising Representation in Clinical Trials: An Interview with Liz Beatty, Inato
The COVID-19 pandemic created a significant shortage of suitable patients for clinical trials worldwide. Within this crisis was another more serious issue around inclusion and representation, driven by COVID’s disparate effect on certain ethnicities, that spoke to a wider issue...
4 years ago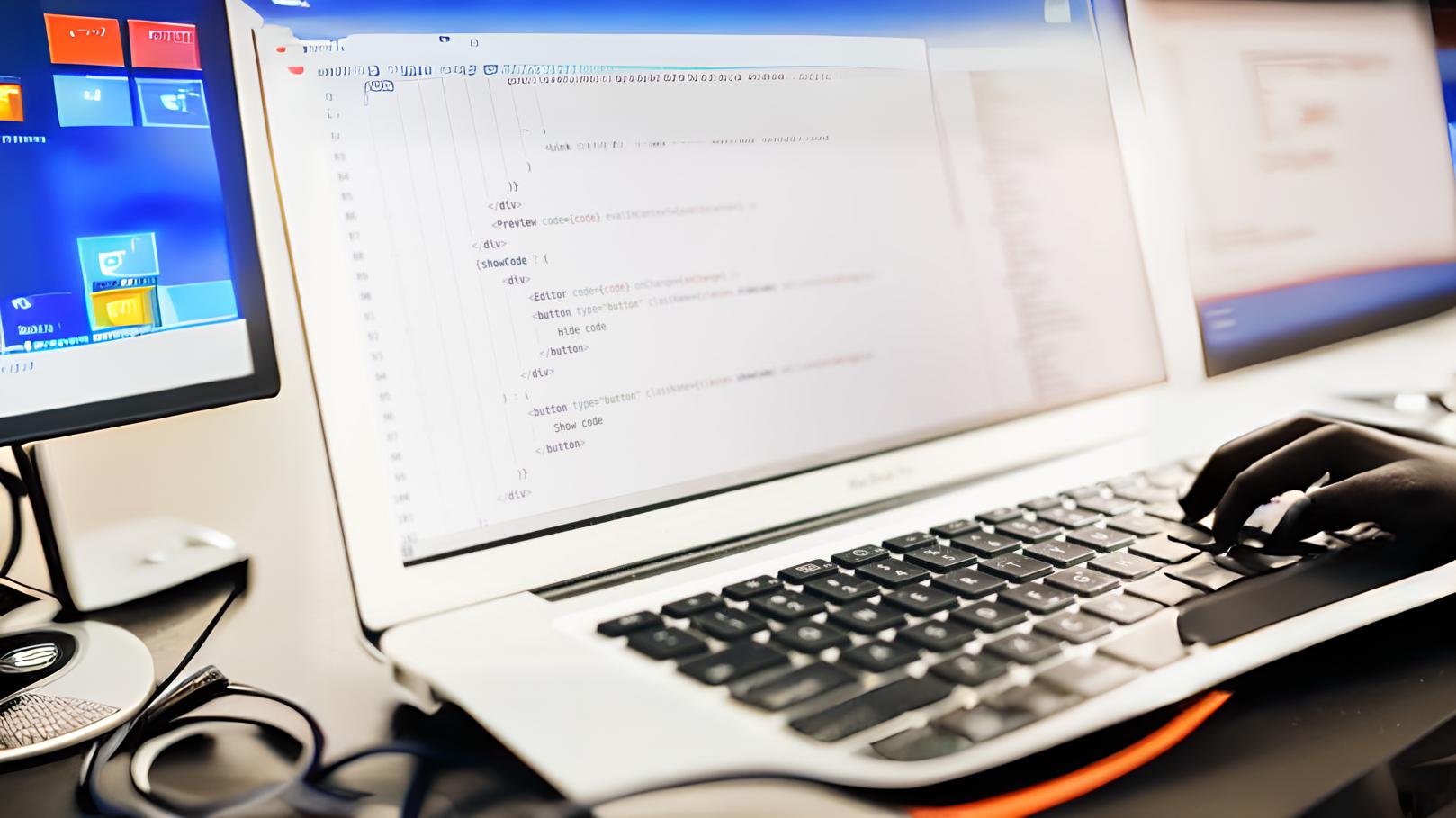
Utilising Machine Learning in Drug Discovery: Opportunities and Challenges
From artificial neural networks to predictive modelling, artificial intelligence (AI) is making its mark in the pharmaceutical industry. The ever-increasing cost of development, shorter timelines and rise in demand has seen the industry reach out to AI to help bring...
4 years agoUtilising Machine Learning in Drug Discovery: Opportunities and Challenges
From artificial neural networks to predictive modelling, artificial intelligence (AI) is making its mark in the pharmaceutical industry. The ever-increasing cost of development, shorter timelines and rise in demand has seen the industry reach out to AI to help bring...
4 years ago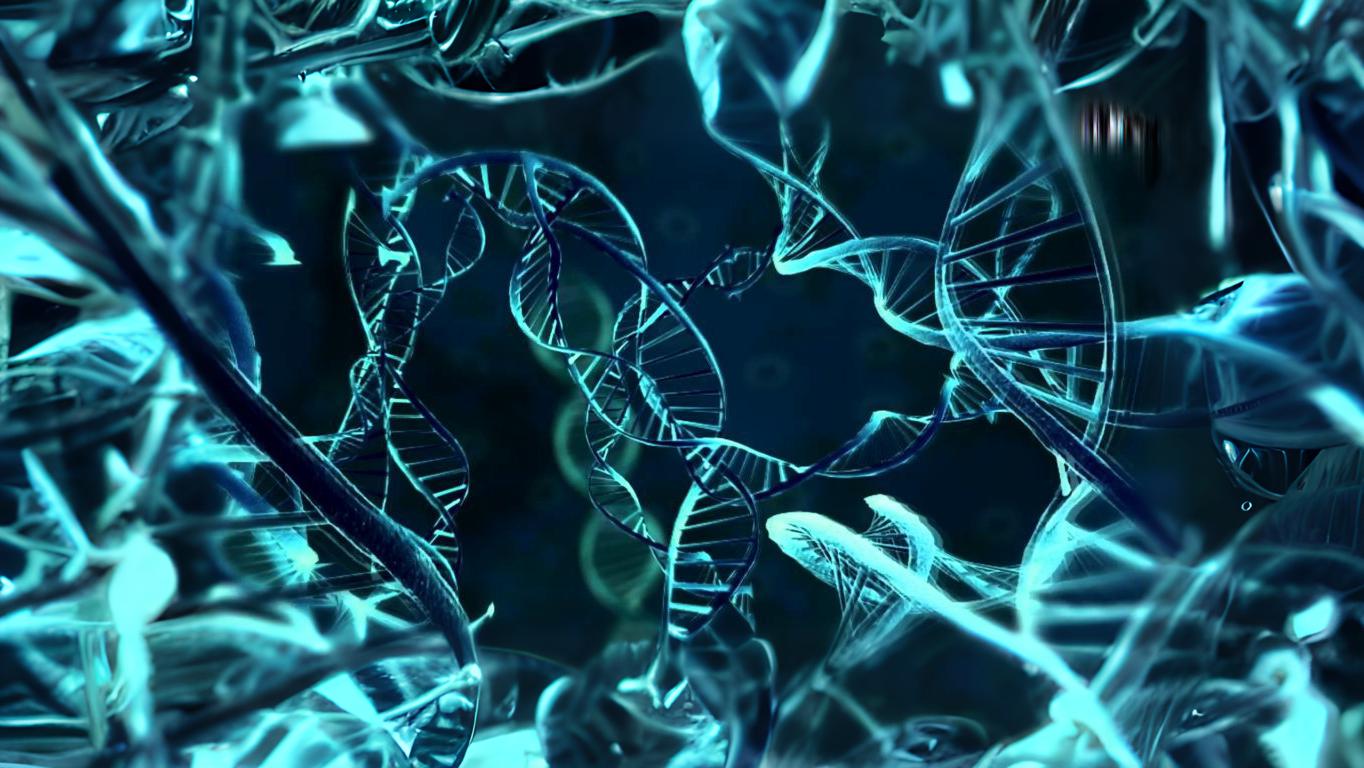
Using Translational Research to Bridge the Gap Between Preclinical and Clinical Research
The disparity between animal models and clinical efficacy is compromising the safety and efficacy of therapeutic drugs. Translational research is building the bridge between preclinical studies and therapeutic treatment. Genomic techniques and non-traditional animal models are examples of translational approaches...
4 years agoUsing Translational Research to Bridge the Gap Between Preclinical and Clinical Research
The disparity between animal models and clinical efficacy is compromising the safety and efficacy of therapeutic drugs. Translational research is building the bridge between preclinical studies and therapeutic treatment. Genomic techniques and non-traditional animal models are examples of translational approaches...
4 years ago