Utilising Machine Learning in Drug Discovery: Opportunities and Challenges
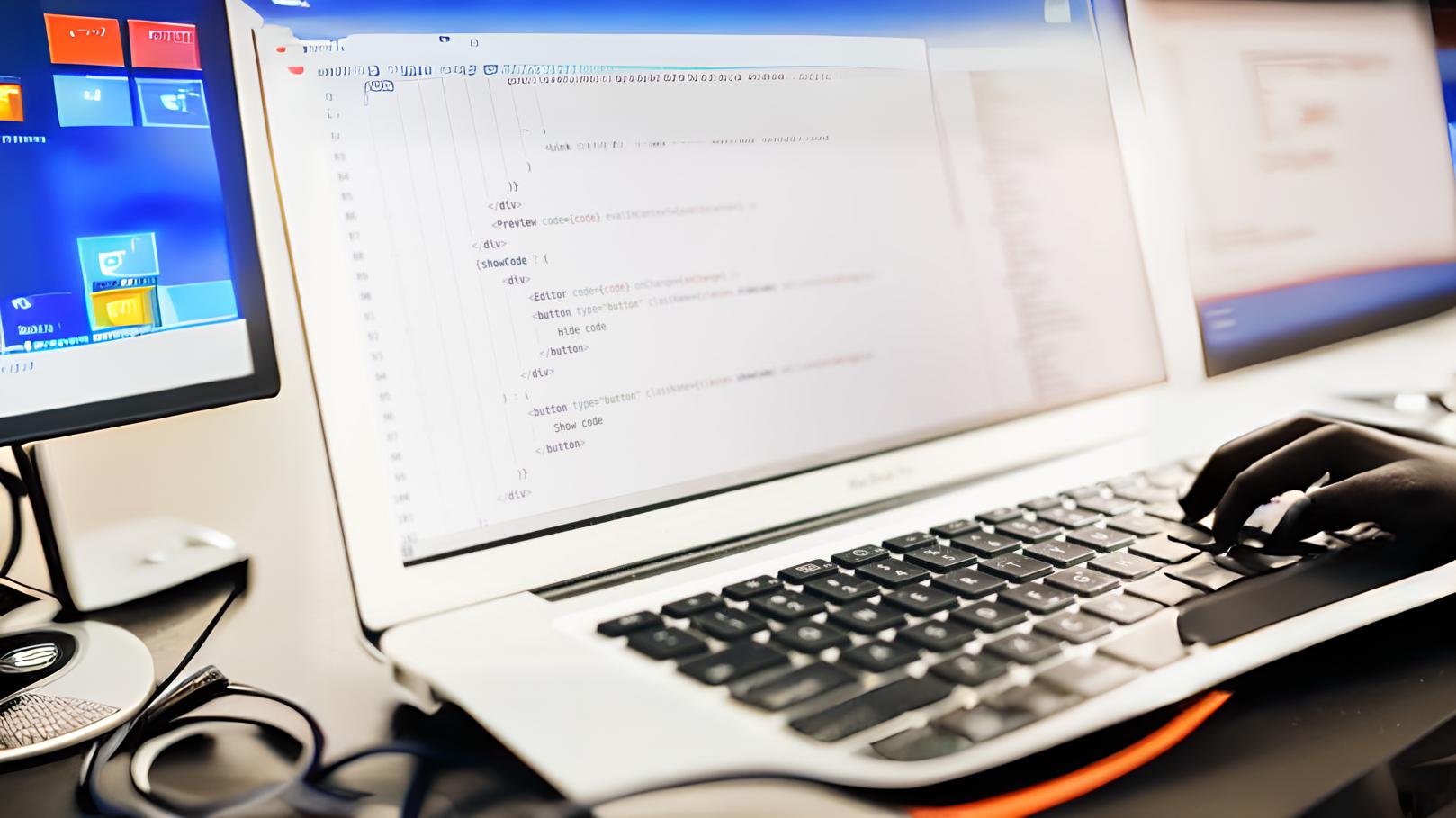
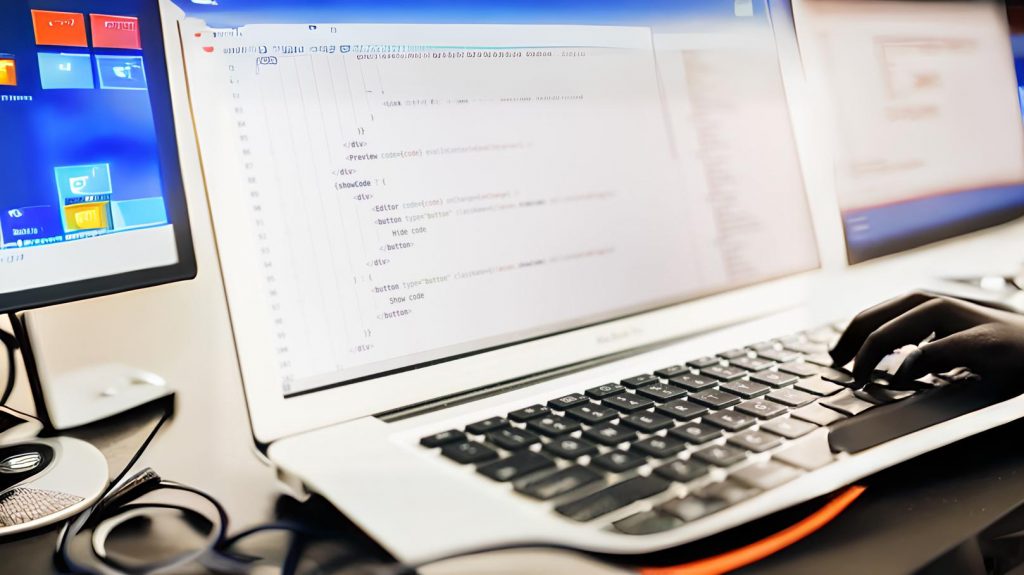
From artificial neural networks to predictive modelling, artificial intelligence (AI) is making its mark in the pharmaceutical industry. The ever-increasing cost of development, shorter timelines and rise in demand has seen the industry reach out to AI to help bring drugs to market faster and cheaper. Machine learning (ML) is one branch of AI with exciting applications in drug discovery.
For daily articles on the latest pharma trends and innovations, as well as interviews with leading experts and in-depth industry White Papers, subscribe to PharmaFeatures.com.
The cost of bringing a drug to market continues to increase at an exponential rate. According to a recent analysis, between 2009 and 2018, US biopharmaceutical companies spent approximately $1 billion bringing each new drug to market. The majority of large expenses occur during the early phases of drug development in drug discovery
Hear from some of the industry leaders including Huijun Wang – who will be providing her expertise in leading a discussion Expediting the Drug Discovery Process Through Chemistry and Biology: Leveraging AI in Hit Finding and Lead Optimisation. To discuss these innovations and more with other leading experts in an informal setting, sign up to Proventa’s Medicinal Chemistry and Biology Strategy Meetings, held online on 29 June 2021.
Drug discovery
Human dose production is a particularly challenging area, in which conventional in vitro modelling faces a dilemma with poor translatability. This often results in early termination of clinical trials when drugs do not demonstrate the same pharmacokinetics within humans as predicted preclinically.
It appears that ML, a branch of AI, is leading the way in the latest innovations. A recent example was a 2021 study which used ML attempts for predicting human subcutaneous bioavailability of monoclonal antibodies. The measured bioavailability of the monoclonal antibodies ranged from 35% to 90%. The decision tree-based method, a form of ML, proved to best predict bioavailability.
Since all of the ML approaches used theoretical calculations and predictions for input, it was suggested from the study that these models may be most useful for early-stage activities like molecule formational design.
A form of AI known as natural language processing (NLP), can be used to optimise the process of target identification. NLP extracts “meaning from human language to make decisions based on the information”. This can be used to scan vast numbers of publications and genetic databases to search for gene-disease associations and identify new targets. The AI-based algorithms can perform tasks such as this with greater accuracy and speed in comparison with human intelligence.
The importance of prioritising the most potent compounds for a relevant therapeutic target is emphasised in a 2018 study investigating machine learning for predicting drug-target interactions.
In the publication, it is emphasised that hit identification “is the first step towards new drug development. Identifying unexpected off-targets can open the possibility of drug repurposing or can lead to insights for predicting and explaining observed side-effects.”
Machine learning on DNA-encoded libraries
DNA-encoded libraries (DELs) have been increasingly explored in recent years to enhance hit identification in drug discovery. DELs represent a modern and versatile tool used to better identify a greater range of novel biological compounds. These libraries are capable of screening drug targets with an extensive number of compounds with great efficiency.
Unfortunately, in order to analyse vast amounts of data, DELs are still dependent on bioinformatics operated by humans. The result of this “limits the scale of molecules considered, introduces bias, and makes it difficult to fully utilise the subtle patterns in the DEL selections”.
Utilising ML allows the identification of important features and obvious patterns from a small dataset and uses the information to create projections for larger datasets. In a recent study, two types of ML models were trained on the DEL selection data to classify compounds: random forest and graph convolutional neural network (GCNN).
The random forest is an algorithm that creates a predictive model comprising a large number of individual ‘decision trees’ which operate as a whole group. Each tree in the forest produces a class prediction and the class with the most votes becomes the model’s prediction.
These methods have already demonstrated success in a study which reported that ML models verified hits up to 29% at one micromolar. The ability to identify target molecules on a micro scale is critical for creating a larger hit pool.
GCNN is a form of ML known as deep learning. One of the main benefits for the application of GCNN for DEL is that “deep learning methods automatically extract important features from a dataset whereas manually generated features are necessary for conventional machine learning algorithms”. Therefore, GCNN is more likely to identify potential hits faster and with greater accuracy than DEL alone.
Iterative screening
High throughput screening (HTS) is the most popular approach for screening large libraries of compounds against a target of interest. Unfortunately, the sheer size of these libraries results in a high screening cost to run. In addition, the low hit rate of HTS, typically less than 1% in most assays, requires large compound libraries to generate a sufficient number of hits for drug development programs to progress.
Iterative screening is a process in which drug screening is performed in batches – each batch is filled by using ML to select the most promising compounds from the library based on the previous results. Iterative screening has been shown to enhance the efficiency of HTS, as it allows for a smaller part of the library to be screened at a time, while still identifying a large portion of the active compounds.
Previously, an iterative approach to HTS was considered impractical due to the high labour costs, however “advances in screening automation have made custom selection of compounds more broadly feasible”.
A recent study investigated the iterative approach to HTS, and found that “the hit rate in the iterative screening was just greater than twice that of normal (random) screening, recovering a median of 78% of the active compounds when 35% of the library had been screened”.
It is worth noting that there are a number of potential practical challenges that can arise with iterative drug screening. While the iterative approach to screening increases the rate of hit identification, the overall process can be “resource intensive and the interim analysis of screening data will potentially require more time for quality control and data management”. These are considerations that will need to be taken into account when weighing up the value of hit identification and whether these challenges can be overcome in the future.
The results from this study however, remain positive and demonstrate how ML approaches like iterative screening show potential to optimise drug discovery.
To discuss these topics further with sector experts, and to ensure you remain up-to-date on the latest in clinical development, sign up for Proventa International’s Medicinal Chemistry and Biology Strategy Meeting, set for 29 June 2021.
Charlotte Di Salvo, Lead Medical Writer
PharmaFeatures
For more articles covering the pharmaceutical industry, clinical research and academia, visit our content site PharmaFeatures.
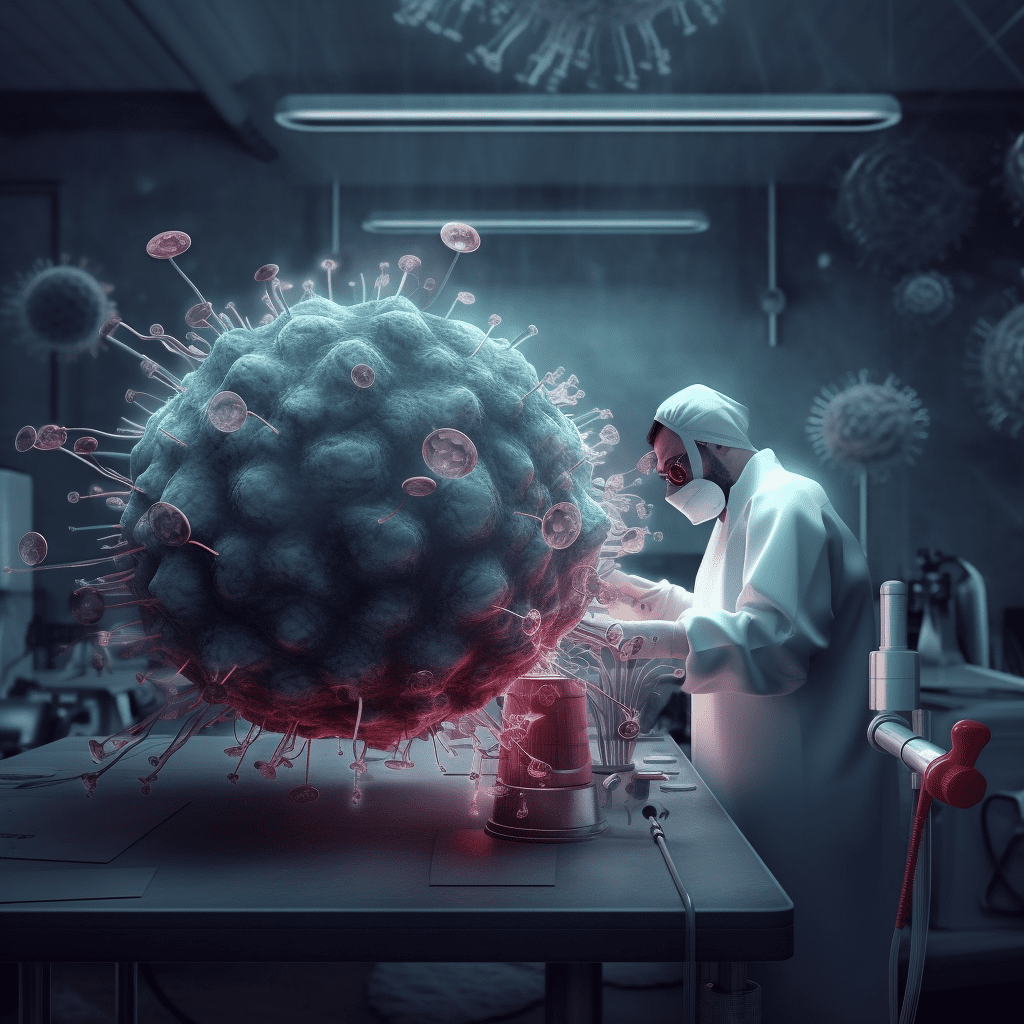
Role of an Immunologist: Exploring the Responsibilities
In the intricate web of human health, the immune system stands as a vigilant guardian, tirelessly defending against potential threats. At the forefront of understanding, deciphering, and harnessing the immune system's power is the immunologist. This article delves into the...
12 months agoRole of an Immunologist: Exploring the Responsibilities
In the intricate web of human health, the immune system stands as a vigilant guardian, tirelessly defending against potential threats. At the forefront of understanding, deciphering, and harnessing the immune system's power is the immunologist. This article delves into the...
12 months ago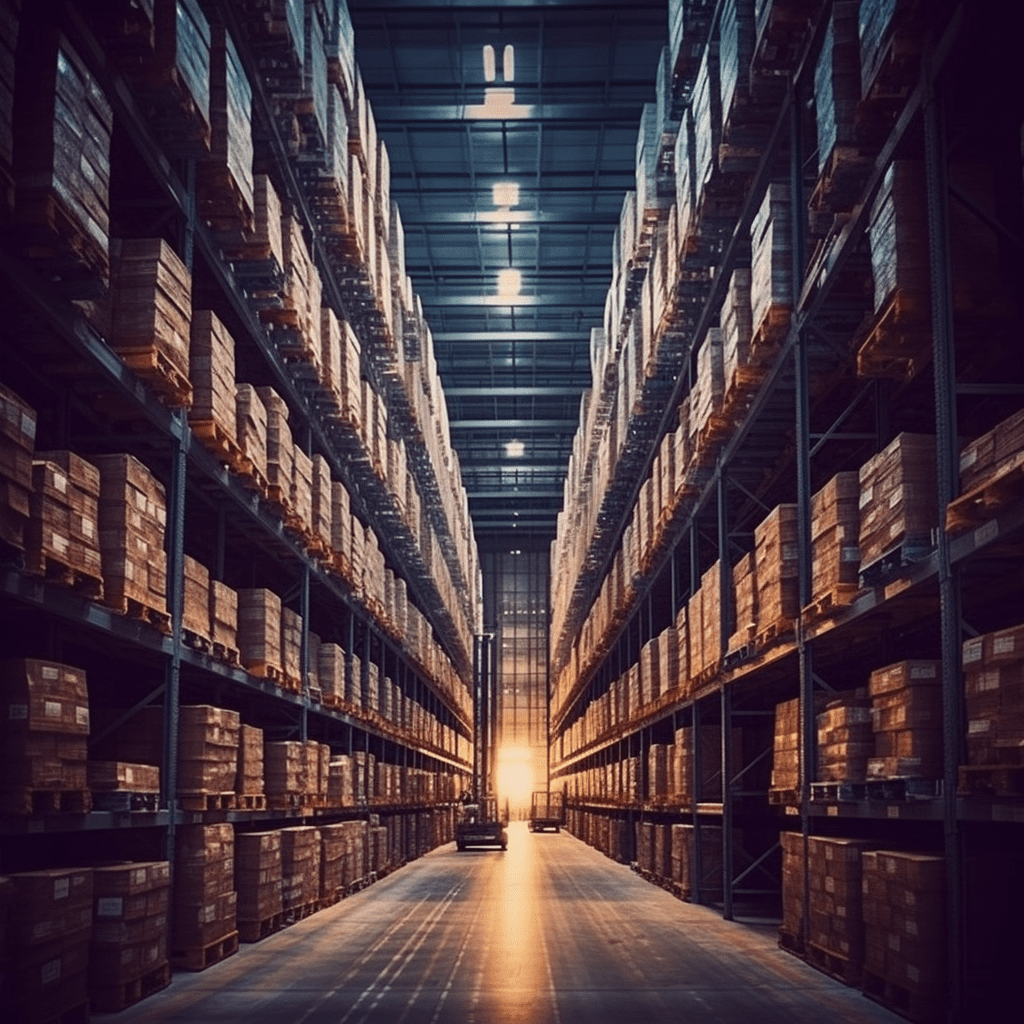
Improving Warehouse Efficiency: The Role of Technology and Best Practices
In the fast-paced world of supply chain management, warehouse efficiency plays a pivotal role in ensuring timely and accurate order fulfillment while minimizing costs. With the rising demands of e-commerce and globalization, businesses are continually seeking innovative ways to optimize...
1 year agoImproving Warehouse Efficiency: The Role of Technology and Best Practices
In the fast-paced world of supply chain management, warehouse efficiency plays a pivotal role in ensuring timely and accurate order fulfillment while minimizing costs. With the rising demands of e-commerce and globalization, businesses are continually seeking innovative ways to optimize...
1 year ago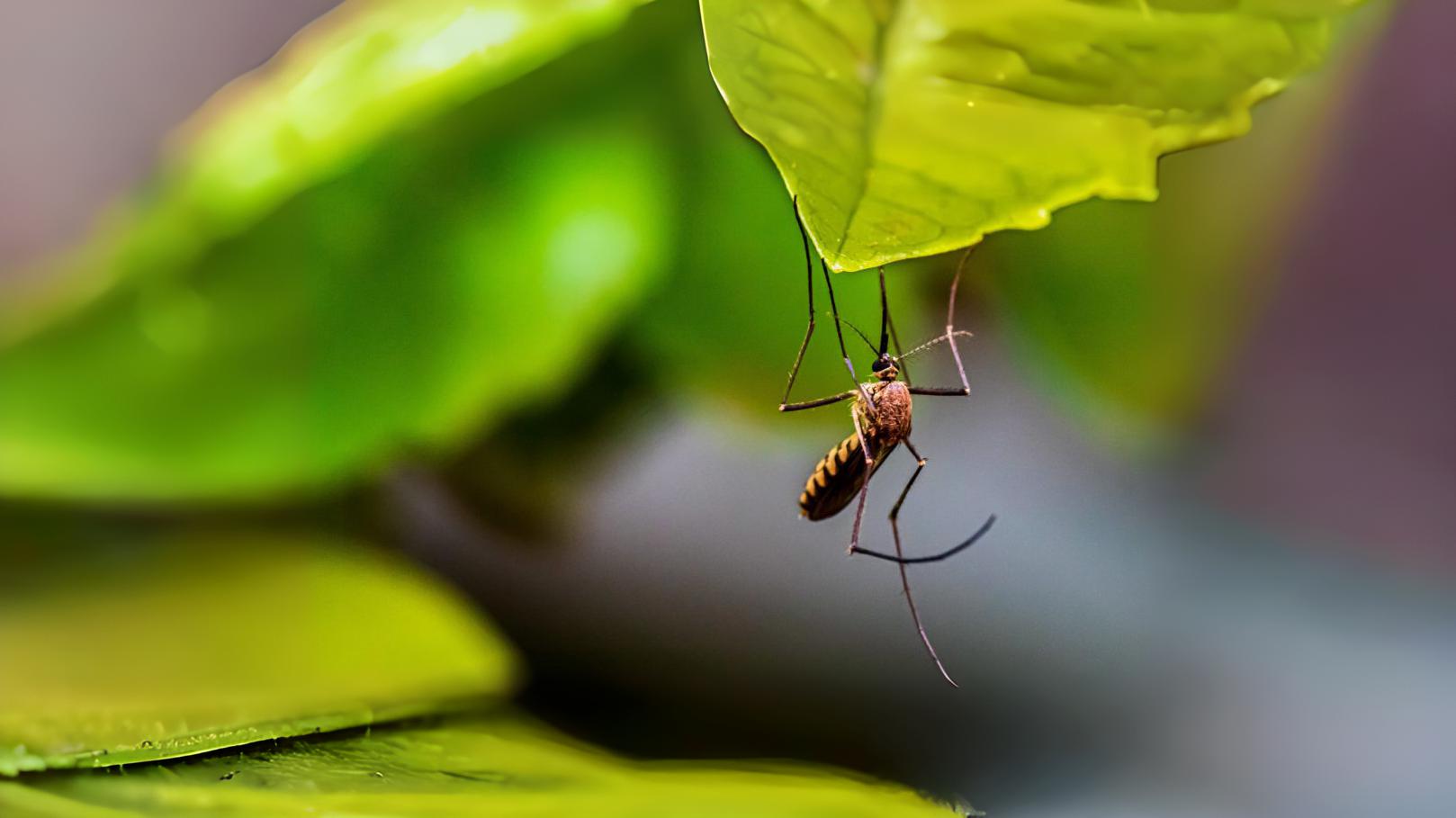
RNA Therapeutics: The World’s First mRNA Malaria Vaccine
Malaria represents one of the greatest unmet clinical needs in the world. In the last decade, research has been ongoing to develop effective preventative methods and effective treatment to reduce the risk of severe illness, which typically occurs in children....
3 years agoRNA Therapeutics: The World’s First mRNA Malaria Vaccine
Malaria represents one of the greatest unmet clinical needs in the world. In the last decade, research has been ongoing to develop effective preventative methods and effective treatment to reduce the risk of severe illness, which typically occurs in children....
3 years ago